Upon the release of the Copilot Studio Kit by Power CAT, AIS took the initiative to explore and configure the solution. We held weekly workgroup calls to delve into the kit’s capabilities, also known as the accelerator. The kit assists in testing custom copilots and uses large language models (LLMs) to validate AI-generated content from these copilots. You can configure copilots and test sets. The kit consists of a packaged managed solution with various components, including a model-driven app, flows, and plugins, to name a few.
Representation from various solution areas attended the exploration sessions, sparking insightful discussions. To our surprise, our CTO participated in the first meeting and was eager to learn more. Initially, we felt anxious, thinking attendees would be expecting a fully figured-out presentation, but quickly felt at ease as everyone was just excited to explore the tool.
The first session focused on analyzing the solution’s components in detail. The participants configured the studio kit for their environments to provide further feedback.
A team member working with a current customer brought their real use case which involved Copilot Studio and Azure Open AI to test with the accelerator. We conducted functional testing of the studio kit using the copilot. We concluded that the setup and testing experience was lacking and there is room for improvement. Here are some of the team’s observations:
- The user interface of the kit is in line with other solutions like the COE kit, making it intuitive.
- There was some trial and error involved in configuring the studio kit with the copilot. Additional steps were needed, and the kit could benefit from better documentation.
- The kit was less useful than the native ‘Test the Copilot’ conversation map feature in Copilot Studio.
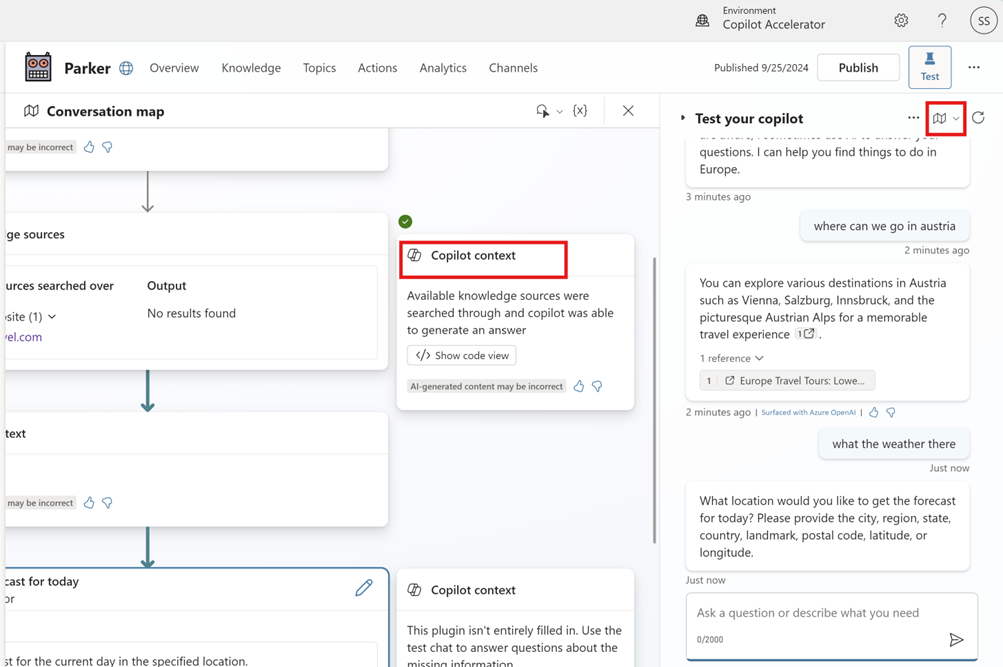
Changing the course of exploration
The hands-on experience with the kit generated innovative ideas for new applications and use cases, extending beyond the initial scope of the meetups. During the configuration process, the team uncovered lesser-known features that significantly enhanced the custom copilot, such as advanced data connectors and custom workflow automation.
Copilot Studio has impacted various solution areas across our organization. Unlike Power Virtual Agents, which was closely tied to the Power Platform. Our Data, AI/ML, and Modern Work areas use the tool widely.
We explored prompts, set up skills, and configured approval flows from the agent. We deployed conversational AI chatbots across various platforms, including websites, Teams, Slack, and Skype. This enabled us to evaluate Studio’s engine and emerging generative AI features.
Learnings from the series
- Understanding your audience i.e. the end user is paramount while designing a chatbot.
- Craft precise and clear prompts. Ensure your instructions are specific to avoid ambiguity and help Copilot provide precise answers.
- Customizing responses and maintaining conversational context for natural conversations is challenging with Studio’s out-of-the-box features.
- We encountered limitations and hiccups while trying to achieve fluid and comprehensive responses. Extending Copilot Studio’s native capabilities with Azure OpenAI integration enhances generative AI responses and improves conversational flow.
- Privacy and security are critical when handling sensitive data, and the possibility of adopting federated authentication was discussed.
- While the studio offers a no-code graphical interface, there is a learning curve associated with effectively using it.
- Participants gained an understanding of the differences between Microsoft 365 Copilot, Copilot Studio, and Azure OpenAI integration, leading to various new engagements.
- The discovery meetings fostered unexpected collaboration between departments, leading to breaking silos. We made new friendships!
- The learning journey became a fun and engaging experience, boosting team morale and fostering a culture of continuous learning and collaboration, which is native to AIS.